I recently had the pleasure of sitting down with Dr. Khai Minh Pham, a visionary at the intersection of life science and artificial intelligence. While AI has only recently entered the public conscious, Khai has been a trailblazer in the AI space, starting with growing and exiting a groundbreaking AI company, DataMind/RightPoint, in 2000s.
His latest company, ThinkingNode Life Science, is both truly innovative and terribly interesting – he’s working on revolutionizing the way we approach healthcare and drug development by running both through the lens of AI-generated digital cell clones.
We went in-depth on a number of subjects ranging from Philosophy to biology, covering topics like the types of knowledge, reasoning vs pattern recognition (and what that means for AI), how Khai started his first company without even a computer or money in France, and, in the end, how technology has the capacity to allow us to truly become more human.
If you’d like to learn more, you can reach Khai on his LinkedIn.
I’m primarily interested in the concept of resilience – whether personal, communal, or societal. What does the concept of resilience mean to you?
In the business world, people often say that entrepreneurs take more risks. I don’t see it that way – or, I guess I don’t see risk the same way as most people. If I did, I don’t think I would do anything! I’m not even sure that you have to do anything specific to be resilient – you just do things, and let other people qualify you as resilient. It’s more about the end result, and the process of getting to that end result, than anything specific that you’re doing.
I find that business, and entrepreneurship, is like a long journey. You take it one step at a time. You just have to be motivated to get to the very next step. And then, at the end, you look back and realize, wow, I’ve done all that?
Perhaps continuously finding that motivation to just get to that next step over and over is what you would call resilience.
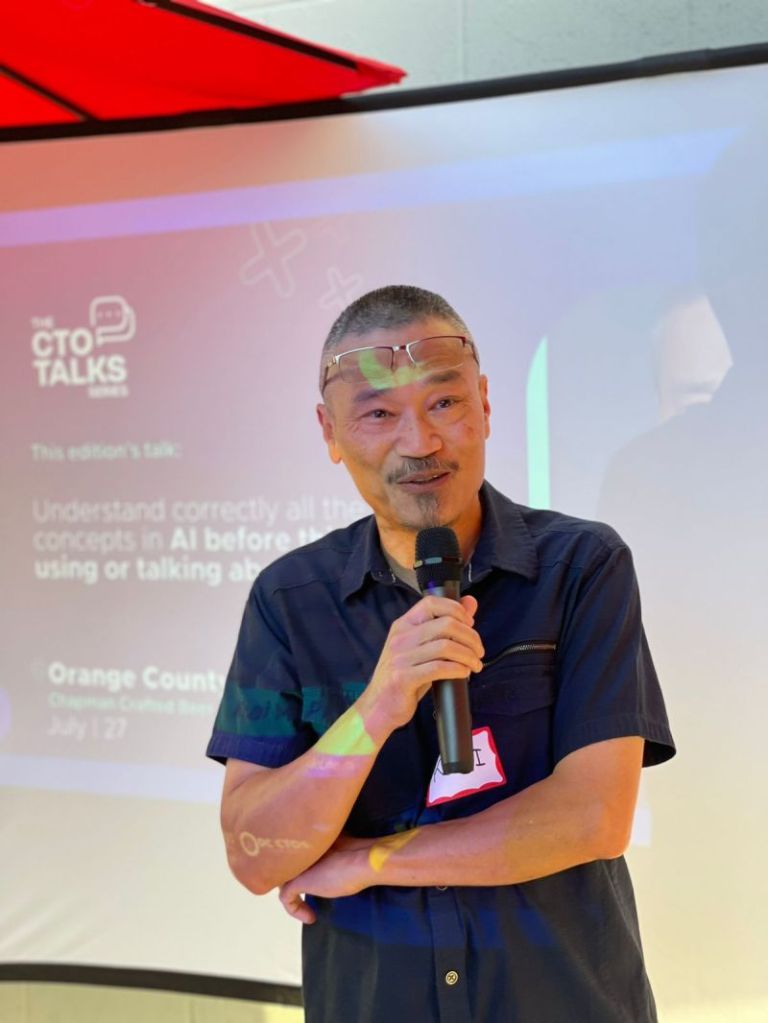
You’ve had an interesting career journey – while a buzzword as of late, you’ve actually been at the forefront of AI for 30 years. In the 90s, you founded the company DataMind, which was an AI platform for the Fintech/CRM industry, and guided it to a $630 million exit. Can you talk about your journey as an entrepreneur?
My mom is Vietnamese, so I always say I didn’t have a choice on what I would be when I grew up – I had to be a physician!
I entered medical school in France, but in my second year of the program, I realized that there was no way that I could remember all of this information. I couldn’t be sure that I wouldn’t forget anything when I diagnose or treat a patient – but I wondered if AI could do it. But when I talked to the AI people, their explanations of what AI was actually capable of was not very satisfying – so I decided to work out for myself the way AI could solve my problem.
Most people in AI would design a formal system, and then try and fit the way we think into that system. I did the opposite. I had a problem, which was how to handle the different types of thinking that you find in medicine, and I worked to design a system that could reason. So, in the mornings, I would head to the hospital, but in the afternoons, I would go do computer science, and in the evening I would catch up on my medical studies. I was a real nerd already teaching AI and working for the company.
Once I did my PhD in AI, I started to publish in main peer-review systems, such as IEEE. It was frustrating since I realized that once I got my grants, that was it – there weren’t enough resources to develop the type of AI I actually wanted to develop. I decided then to start my first company in order to have get enough resources to do what I wanted to do. I didn’t have money, I didn’t have a computer. But I decided just to go for it since I had such a desire to do this research. It started simply because I wasn’t satisfied with what existed.
Wait a minute, so you started your first company without a computer?
Well, I didn’t have a computer and I didn’t have any money either! Actually, it was the other way around – I didn’t have money, so I couldn’t afford a computer!
So my first goal was to get a computer. At that time, Sony was providing workstations if you had an interesting project. I went in there, gave my pitch, and they gave me two! But then I had a different problem, because now I had two workstations but I didn’t have a desk. So I went to a meeting in France for entrepreneurs and I met a few of them. I told them that AI was going to be important one day – and if you give me a desk to work out of, I can explain to you what I’m doing and maybe it will be interesting and helpful for your business down the road. One of them eventually accepted, and I had a desk! So now I have a computer and a desk, but I don’t have people working with me – so I had to head to the bank!
Imagine walking into a French bank at the time to explain AI. Nobody knew what it was. But I believed in my passion so strongly and had such a great technology that eventually I walked out with a credit line, and I was able to hire an engineer and an assistant. I then took my savings and went to a big conference in Silicon Valley. There, I ended up meeting the President of Microsoft Europe. I told him that he had nothing left to prove at Microsoft and asked him to come join me! Again, I don’t think I’d have done things like that today!
Something I learned very quickly was that people at the top level of business have more freedom to do what they want, while the intermediate level has to produce – because a few months later that Microsoft exec was in my office and we were talking about the technology!
Then, one day, I was out to lunch and when I came back, my assistant said that I had missed a call from a “Mr. Gatess.” (with two s, since she is Portuguese and had not been in the computer business). I didn’t know who that was – but then I realized that it was actually “Mr. Gates!” She didn’t take his phone number down, so I was really hoping that he would call back – luckily, he did! So I had a meeting with Bill and we talked about AI and the tech.
The main thing that you realize when you work with a big corporation is that their time is not the same as your time. They have all the time in the world, and they want to use it to understand everything they can. After the meeting with Gates, he set up a meeting with the CTO of Microsoft at the time, Nathan Myhrvold. But I realized that I didn’t have the resources to do what they wanted yet, so I had to decline. They even offered me to join Microsoft and work on the research I wanted and wouldn’t need to think about the budget.
Then, somehow, IBM learned about my company and they wanted to invest. But they were too big for me. Then the VCs found out about my company, and that’s how I ended up really starting my own company in Silicon Valley. After that, everything went very quickly!
I learned too, just how important culture is. When I arrived, I didn’t speak English well at all. I had to translate everything that was said in every meeting into French in my mind. It was exhausting! The way people worked over here was different too – so I had to learn that as well.
So I don’t necessarily think that I’m resilient, but I just kept going and hanging in there. I was just so motivated by this project that it kept the engine going.
That’s an incredible story!
Well, now that I look back on it, I would never do it that way today! For example, when I started, I had never even heard of the term business plan! But I just never stopped. It was really based on an inner motivation. I don’t really feel resilient internally, but from an external viewpoint…maybe I am.
You’ve been at the forefront of AI for decades, at a time when most people are just becoming aware of AI and its capabilities. Can you talk about your own philosophy of AI learning and how that might differ from some of the major players, like ChatGPT?
We could spend hours and days talking about this!
The first thing you have to understand is the difference between data and knowledge. Humans don’t process data. We’re actually extremely bad at it! We process knowledge instead. Data is an isolated fact, but if you relate that fact to other facts, that becomes knowledge. And there are actually two steps for knowledge – information and knowledge. Things move from information to knowledge once it becomes an internal asset for your brain. For example, books are full of information. But that information becomes knowledge once you read, digest, and understand them. This is actually a very important distinction, because as soon as something becomes knowledge, then you can apply reasoning to it.
Most of the time when people talk about knowledge, they actually talk more about information that’s available – and there’s a lot of it. But that’s totally useless until someone acquires it and can reason with it. So that’s the first thing.
The second thing is that correlation is not causation. Correlation allows us to narrow down what’s going on, but causation is what we’re looking for. It’s what science is about, understanding cause and effect, right? For example, I can tell you that there’s a big correlation between people who see a doctor and people who die, but what does that mean?
In most of the AI that people focus on today, it’s about correlation. It’s based on statistical analysis that extracts patterns from large amounts of data.
Why we process data is to generate that knowledge, like I said earlier. Knowledge is the most powerful way to compress data because it lets us do reasoning afterwards.
So when people think about this, you have pattern recognition, which is data driven, and we have reasoning, which is knowledge driven. In AI, most of the AI today is Machine Learning (ML) – detecting and recognizing patterns. And it’s amazing what pattern recognition can do. It’s surprised a lot of people, including me, but it still doesn’t reason the way that humans reason. It’s missing an internal representation of the world that allows it to reason. It’s missing a mental model. ChatGPT makes associations of words, and the result is impressive when you read the results, but can present hallucinations because it doesn’t reason at all.
This last point is crucial. We don’t have access to the real world – we only see the world through our different mental models, and we have different types. The most powerful model, though, is our reasoning model. People studying science are essentially working to build reasoning models for their specific domains. So today, when we use machine learning for science applications, it’s fantastic because it can crunch so much data to narrow down and recognize patterns. But your ultimate goal should be to have a reasoning model at scale because no one can have all the available knowledge – except AI.
This brings us to what we do at ThinkingNode Life Science. We use distributed reasoning AI to generate reasoning models that we call reasoning networks. So we don’t generate text, or images, or videos like ChatGPT – we generate reasoning models for life science. We generate digital cell clones. Another important term is that we use “distributed reasoning,” meaning that we have more than one reasoning engine. For example, our AI reasons by analogy, by constraint, by case, by probability, and so on. If you only have one reasoning engine, if you’re centralized reasoning, it’s not possible to represent all these different types of reasoning. And this distributed reasoning AI is what I’ve been working on for decades now.
So we take our distributed reasoning AI and we use generative AI to generate these digital cell clones. We generate about 50 million additional data points for each digital clone using reason and not pattern recognition. Why do we do that? Well, most AI drug companies and pharma companies are focused on developing and designing new drugs. We don’t do anything related to that. We design and generate the digital cell clone of the patient or the disease to understand the impact of the drug on the cell. We are focused on cell response. I think that’s what matters most at the end of the day – the interaction between a drug and a cell. So we’re not in competition with any AI drug discovery company. We focus on biology, which is the cell – and how cells respond to drugs. We have a patent for digital cell differentiation that allows these cells to scale, and we use the gene expression data of the cell. We inject that into our human cell reasoning foundation model, and it differentiates the stem cells and things for us. So today, in about two hours, we can generate any type of human cell digitally.
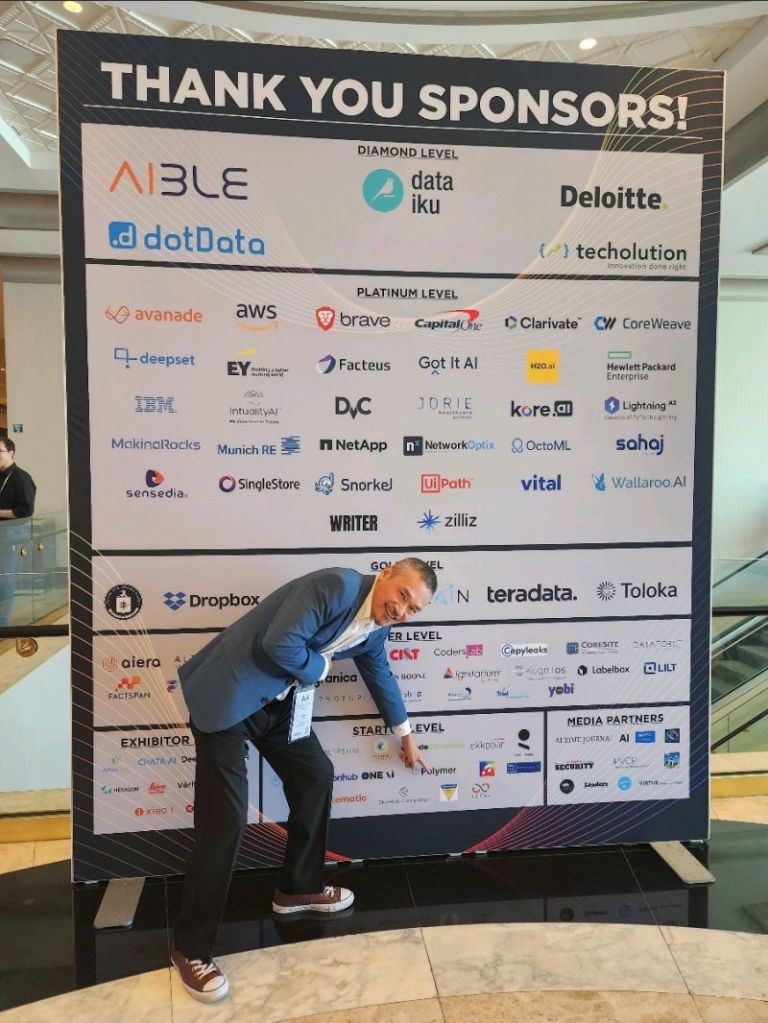
Wow. So, just to restate this in a sentence, ThinkingNodeLife creates digital clones of human cells. You then use those clones, and artificial intelligence, to test and to explore drug interactions on the human body without using a live person. Is that correct?
Yes! It takes about 10 years and $2.6 billion to test a drug, and you still only have about a 4% chance of putting it on the market. There are lots of reasons why, but one of the main reasons is that there is no testing simulation.
In other industries, say the car or airplane industry, you don’t build the car or plane right away. You build a digital model and do simulations. We don’t have that in pharma.
The term clone is important here too – it’s not a digital twin. Digital twins, you have to build one by one. With our clones, it’s totally scalable since we digitally mimic the cell differentiation process. We have a foundation model, and differentiate based on that.
So it seems that ThinkingNodeLife has resilience baked into it – helping the healthcare and biotech spheres shorten drug times, understanding the interactions between cells, and allow companies to help people much faster than before. Can you talk more about how you help these companies build resilience in their workflows?
So we work with different types of companies. At a recent event at JP Morgan, we announced a strategic partnership with Debiopharm. It’s a Swiss pharma company, well established and well respected. They’re going to use our digital cell clone for cancer drug development. So this is one type of customer and partner – the biotech pharma that develops the drug.
With those companies, we can help them at the very beginning – finding new targets for the drug. Usually, this is done by the academics since it takes lots of time. Then, if you already have the drug, even before preclinical testing, we can simulate the drug’s impact on the human cell that you’re interested in. Then, if you’re already in phase 1 or phase 2 testing, we can use a patient’s data to generate their own clones to help companies select the next patient for their clinical trial.
And we can use all that to do different things. We can do drug comparison – comparing your drug to a competitor’s drug – which is normally pretty difficult, because you normally don’t have your competitor’s patient data. We can do drug repurposing and drug response prediction. Once you have all that, you can really help a company become first class, since we provide targets and can simulate all the phases for testing.
Then, we have a different type of company partner – AI drug companies. We don’t develop anything related to the drug, but we do know the cells that drug will impact – so there’s a complementarity there. We’re currently in discussions with a number of them.
We also work with CRO companies outsourcing the research for pharma biotech companies. We bring them Digital Cell Labs, so they can provide research services in preclinical or after clinical, so they can give more insight to their customers about the drug when it’s in development.
I think any company that develops a drug should do a simulation. And we help them do that.
That makes sense. So, you cut your teeth with your first company in Silicon Valley, but you recently moved to San Diego to be closer to our strong life sciences community. Can you talk about the differences between Silicon Valley and San Diego, especially for startups like yours?
Maybe it’s different today, but when I moved here about 10 years ago, Silicon Valley was much more focused on technology and San Diego was much more focused on science. I was lucky to work with the J. Craig Venter Institute down here, and this helped me really test my idea and work on different concepts. So this is a major pro, I think – the scientific community is extremely strong.
The other thing I found is that people are very collaborative in San Diego. I don’t know what it’s like today, but when I was up in Silicon Valley, it was very competitive. Here, we don’t hesitate to work together.
The last thing is that there are over 100 microbreweries! That’s a great part of San Diego.
Can you talk a little bit about the emerging field of digital biology? What is it? How do you see it contributing to building resilience in the fields of life sciences and healthcare?
Digital biology is a difficult definition, in the sense that as soon as you use a computer to understand biology, it can be considered to be digital biology. But what I have in mind is more about getting to a simulation of biology. There are different ways to do simulations, from mathematical models and so on. The one I’m focusing on is AI simulation, and, in particular, reasoning AI simulation.
This is an important concept but isn’t always easy to explain. It starts from realizing that we interact with the world only through our own mental models – only through our own reasoning model that we acquired at school, through our experiences, whatever. Our reasoning model is how we make sense of the world. Scientific education is essentially about building different reasoning models for different disciplines.
The approach that I have is not a pure mathematical simulation, for example, because humans don’t think through mathematical equations. We think through concepts. We think through conceptual mental models. And the way I approach digital biology is to generate the reasoning models for biology. One of the most important entities in biology is the cell – and that’s why we are focusing on providing and generating a reasoning foundation model of the cell.
What does this mean in terms of application? Well, today there are about 800 AI drug discovery companies, and designing drugs is very important. But what may be even more important at the end is the cell’s response to that drug.
In designing a drug, you try to see how the molecule you’ve designed binds to a target, which is usually a protein in or on the surface of the cell. This binding is very important. You see how well it binds, how specifically it binds.
Then, what’s important to us, is seeing the impact from this drug’s binding – you see the cell’s response. Typically digital biology is about simulating the binding itself in order to understand and design the drug. What we are focusing on is not about the drug – it’s about the cell’s response to the drug.
Interesting.
It’s a multidisciplinary team field, and it’s pretty involved – there are different aspects from computer biology, system biology, AI, and so on. The field is working on things like natural biological phenomena or synthesizing new artificial biological entities. In fact, ultimately, the far vision for our company is to simulate evolution.
Digital biology, the way I see it, gives us the technology to accelerate and even be active in the evolutionary process.
Let’s continue talking about how you see the future. While AI has been around for a while, it’s recently come to the forefront of public consciousness with the emergence of ChatGPT – and it’s changed a lot of people’s relationships with technology. What do you see for the field of AI going forward?
Well, first of all, we have to understand what the term “intelligence” means, since it’s artificial intelligence. I used to mention [Swiss psychologist] Jean Piaget’s definition – intelligence is not what you know, it’s what you do when you don’t know. And I’d add to that – getting to a rational outcome when you don’t know. Since, for example, ChatGPT will provide you an answer, but it cannot explain the why behind the answer. It has answers based on the association of words and not on the rational reasoning process that usually is based on causality and not correlation. It can give a very convincing explanation but ultimately can go wrong with hallucination. It’s not rational.
When we talk about AI, we have two understand two different aspects of it. Daniel Kahneman talks about these aspects in his famous book Thinking, Fast and Slow. The first aspect is System 1 thinking, or pattern recognition. It’s what you do when you recognize a face or a piece of music. It’s very fast. There’s no reasoning.
The second aspect is System 2 thinking. That’s slower. It’s when you have to think rationally, have some hypotheses, deduce things, and so on.
The AI that is known today by the public is pattern recognition, based on Big Data, and it’s amazing what can be done at scale. The other AI that you don’t hear much about is reasoning AI, and that’s because most of existing reasoning AI is based on just one reasoning engine. But there are different types of reasoning. It’s why I worked for decades on that topic, called distributed reasoning AI, where each piece of knowledge is a mini-reasoning engine in itself, and you throw those mini-engines into any logic you want.
The future of AI is the combination of the two types, as we are, right? We do pattern recognition and we do reasoning – but it’s very important that people realize that AI is not just machine learning. It’s not just pattern recognition. It’s not just ChatGPT. It’s much more than that. When we see what we can do with pattern recognition at scale, well, imagine what we can do with reasoning at scale.
Reasoning is important, too. It’s the way that humans make decisions. We don’t process data, we process knowledge. When you think about how AI can potentially interact with humans, yeah, it can be easy to interact with based on pattern recognition and lots of text, that’s certainly one way. But if we want to interact with AI and have it be close to how we think and reason, that’s reasoning AI.
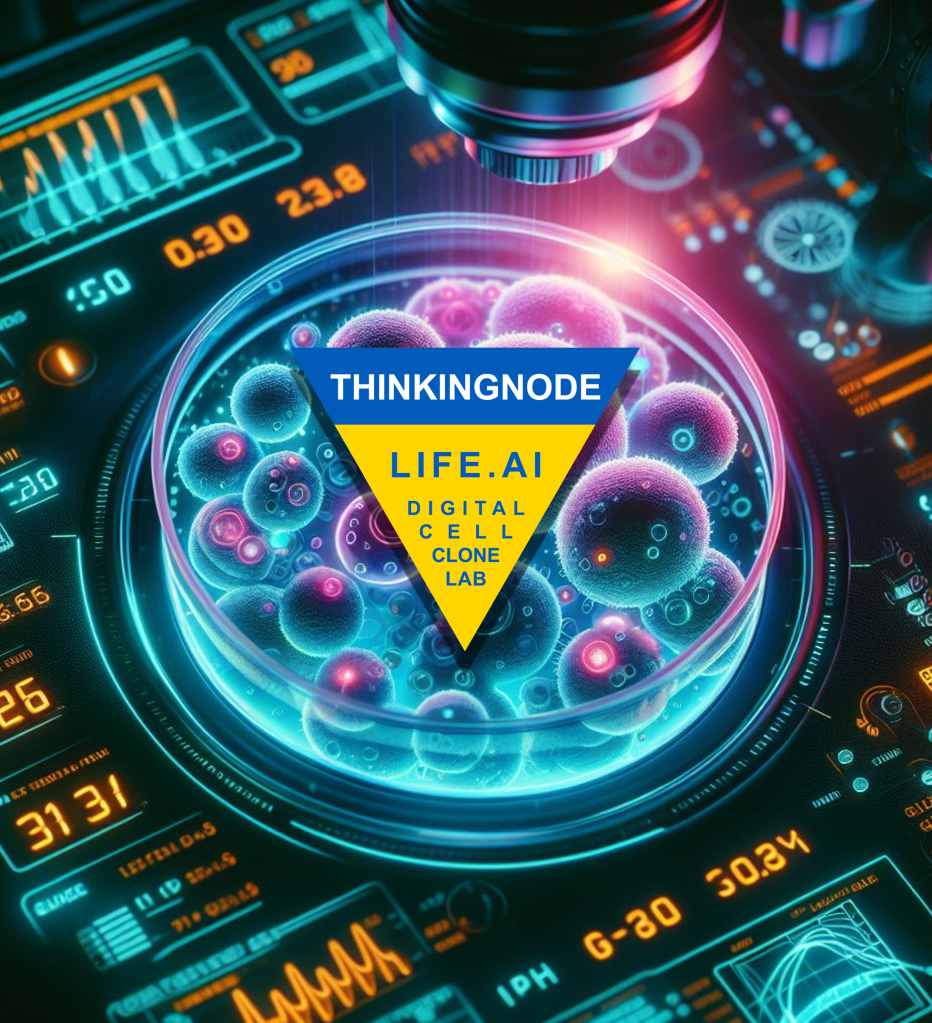
What advice would you give aspiring entrepreneurs and professionals on developing a resilient mindset and making a meaningful impact in the world of AI and life sciences?
As I mentioned at the very beginning of the interview, I don’t know if it’s worth focusing on becoming resilient. What I mean is, resilience is a consequence – it’s a consequence of passion and a consequence of your actions. When you have a passion, you will be resilient. Your goal isn’t to become resilient, your goal was to achieve your passion – but resilience was found on the way.
So I don’t have any tips for resilience, but I have tips about being passionate. It’s about looking backward in your childhood, looking for what is meaningful, and focusing on that. Find your life’s purpose. Applying it to life science now, well, what can be more interesting than life itself? And when combined with AI, what’s more interesting than working on how we reason and understand? When you combine the two, it’s incredible. That’s why I’m so passionate about both. It’s how to understand reasoning, and applying that to improve life.
What’s next for ThinkingNodeLife, and how could somebody who’s reading this blog potentially help you?
We’re currently in the middle of Series A fundraising, and we’re looking for people who are passionate. It’s nice to be smart, but it’s not enough. I want people who are interested and are going to enjoy the journey, because, if you enjoy the journey, you can go very far. We’re looking for people who are open-minded, not people who only want to prove that they’re really smart. We want people who both really understand the mission and are on mission.
So, the next concrete step we’re taking is finding funding, but we’re also focused on finding customers. We have some events coming up, like at the BIO International Conference in June. We’re looking at partnerships too – we just partnered up with Debiopharm, a pharmaceutical company in Switzerland.
And finally, if you’re passionate about AI and life sciences, I’d be happy to meet.
What’s the best way for somebody to contact you, or your company, if they’d like to learn more?
The best way would be LinkedIn. I’d be happy to connect. You can also find my company on LinkedIn too.
Any final thoughts before we go?
People talk a lot about technology, and sometimes people focus too much on the technology side of things. I think what we need to focus on instead is how technology can allow us to be human. I almost don’t consider us to be human yet. When I see what’s going on in the world, we’re pre-human. How can we use our technology to allow us to have more time, to be less greedy, to create abundance?
And what do you think it means to be a human?
Well, that’s a huge question of course. People talk about consciousness and so on, but I think being human is thinking of others, not about yourself. Being human is the ability to contemplate, the ability to appreciate things. Being able to sit and contemplate the beauty of a tree, for example. Animals don’t have the luxury or capacity to do that – they can only think about survival.
Humans though, by thinking of others, have the ability to appreciate, to protect, to build. And leaning into these gifts is what makes us human.
Want to go more in depth? Learn more here:
ThinkingNode Life Science Website
ThinkingNode Life Science LinkedIn
ThinkingNode Life Science – Debiopharm Partnership
Interview with Khai Pham in AIMed
Thinking, Fast and Slow by Daniel Kahneman
Leave a comment